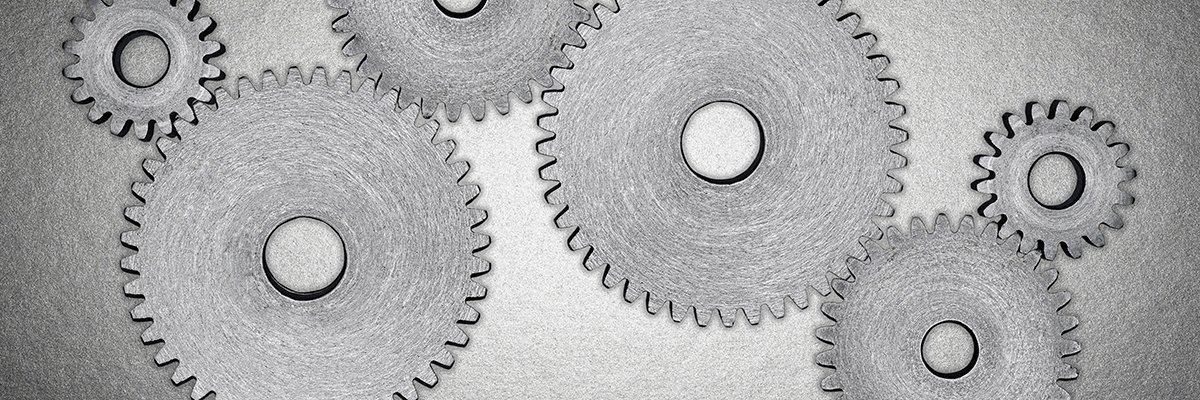
Salesforce senior vice-president of artificial intelligence (AI) Jayesh Govindarajan leads the company’s AI organisation and is responsible for the family of products the software-as-a-service (SaaS) provider builds and serves to its customers.
Govindarajan says Salesforce’s AI customers fall into two broad categories. “There are the customers who desire turnkey AI, which is deeply already integrated into their workflows, and then there are the sales professionals, service professionals and marketing execs.”
For the former – those customers who want to build their own AI – Salesforce’s Einstein platform brings together generative AI (GenAI) and predictive models.
For the latter group of users – those in sales, services and marketing roles – Salesforce has built AI technologies into its product offerings. “In the past, that was predictive AI. Now we’ll bring them into the generative AI world,” says Govindarajan.
As is generally the case for adding AI to enterprise software, Salesforce is targeting efficiency. “What used to take hours of research on a particular company before you reach out with an introductory email can happen in a few seconds,” he adds.
Other efficiency benefits arise when GenAI is combined with existing business process workflows that enable the enterprise system to handle edge use cases, such as exceptions to normal processing that may require human intervention.
Combining GenAI with enterprise workflows
Govindarajan gives an example of how datasets from enterprise systems could be combined with a conversation with a chatbot. He says the chatbot would typically be programmed with a set of responses it needs to make based on a predefined flow chart, but “at runtime, this changes dynamically when I give it a task or an instruction to resolve”.
Govindarajan says such a task requires a combination of contextual data, based on prior knowledge, plus a set of actions that have been registered with the system. The system then needs to orchestrate these in the right order to enable the chatbot to respond to a query. “The flow chart is being created dynamically based on the conversation with the customer,” he says.
Longer-term effect of efficiency gains
Enterprise AI enhancements are designed to improve productivity. While no one is saying this will result in an immediate decline in the number of employees required to do a particular job, long-term commercial pressure is likely to result in a slow decline in the workforce as AI takes hold. The revenue of IT providers like Salesforce may be directly impacted by this decline in the future: if there are fewer employees, a business may not require as large a SaaS subscription.
Govindarajan is not afraid to suggest that price increases may be needed in response. “If the best way to deliver value is to make people 10 times more productive, then we should go off and do that,” he says. “If you’re truly delivering that value, which is making somebody 10 times more effective, people will be willing to pay.”
Near term, he sees the benefit of AI as being that it will free up staff to do higher-value tasks. “We are seeing tremendous amounts of value being created,” he says. “[But] what happens with all the free time you generate?”
Govindarajan sees opportunities to reskill staff. He gives an example in which Salesforce implemented a service centre for a large luxury retailer where staff spent most of their time processing return orders or apologising for an issue with an order. By introducing AI to process the returns, he says: “The staff found they were able to connect a lot more deeply with the customer. What used to be a cost centre for solving tickets suddenly became a profit centre because people are now upselling.”
Losing muscle memory
There are clearly benefits to staff and businesses if people can be moved into higher-value jobs. But there is also a risk that people become less able to perform the tasks they used to do.
With this in mind, Govindarajan believes those developing AI systems should draw on the experience of AI in aircraft. “People who fly planes use autopilot a lot. So you have to be very mindful about designing systems that have what’s called friction, which you put in the system so people don’t forget and can take over when they need to.”
Govindarajan believes software developers will use such techniques in the interfaces they design for their applications. “You can prompt the user to take more action so they don’t [lose the] muscle memory. I’m not saying that this is happening today, but if you look at the airline industry, that’s what they do.”
Built-in friction is also a feature in Tesla Auto Drive. The system provides self-driving functionality, but Govindarajan notes: “You have to keep your hand on the wheel and the interface reminds you to do so.”
The conversation with Govindarajan gives a glimpse of the second-degree effects of AI in the enterprise that business and IT leaders may not have to consider immediately, but are likely to take effect as more AI is embedded in working practices.
“There is a lot of thought around how to make AI useful to people and how to get them to adopt it,” he says.
He recognises the need to ensure enterprises see the value of AI and that people do not simply treat it as a toy: “From the CIOs and CEOs I speak to, I have a conviction that this is the year of AI adoption where customers will start to see a ton of value.”
According to Govindarajan, organisations are now starting to scale out AI deployments. But, he adds: “I think a lot of the second-order effects of AI technologies will need to be thought through and built into the products we use.”
Comentarios recientes